Handwritten offline Hindi character recognition using advanced feature extraction techniques
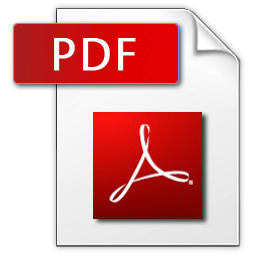
Dayashankar Singh1, J P Saini2, D S Chauhan3
COMPUTER MODELLING & NEW TECHNOLOGIES 2016 20(4) 30-36
1Assistant Professor, Deptt. of CSE MMMUT, Gorakhpur (UP), India
2Professor, Deptt of ECE BIET, Jhansi, India
3Vice-Chancellor GLA University Mathura (UP), India
Feature extraction technique plays an important role in character recognition since last so many years. In this paper, two advanced feature extraction techniques namely 16-Directional Gradient Feature Extraction Technique (16-DGFET) and 24-Directional Gradient Feature Extraction Technique (24- DGFET) have been proposed and implemented. This paper demonstrates the concept of Handwritten Hindi Character Recognition (HCR), feature extraction mechanisms adopted for character recognition starting from Conventional Feature Extraction Technique (CFET), Gradient Feature Extraction Technique (GFET), and Directional Gradient Feature Extraction Technique (DGFET). In DGFET, few techniques have been initiated which involve dividing the gradient values to 8/16 directional values, these techniques attained recognition accuracy of around 94%. We have aimed at further splitting of the gradient values in 24 parts in order to find if it achieves the objective of increasing the performance of character recognition with more accurate analysis and acceptable training time. An experimental evaluation and comparative analysis have been made at the end of the paper to prove the result whether further splitting is providing a better result in comparison to 8 or 16 parts division taking in account the training time, the accuracy of recognition and performance appraisal. The network used here is Multilayer Perceptron (MLP) with Error Back Propagation (EBP) algorithm to train the network.
A sample of count 1000 has been taken for experimentation including the personnel of different age groups involving both male and female handwriting. A comparative synthesis is made for 8/16-Directional and 24-Directional input values comparing the recognition performance and training time.