Faults diagnosis of railway rolling bearing by using time-frequency feature parameters and genetic algorithm neural network
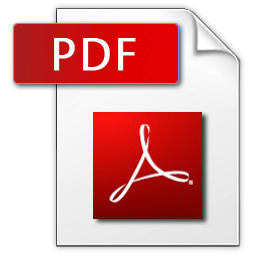
Dechen Yao1, 2, Limin Jia1, Yong Qin1, Jianwei Yang2
1State Key Laboratory of Rail Traffic Control and Safety, Beijing Jiao tong University, Beijing 100044, China
2School of Machine-electricity and Automobile Engineering, Beijing University of Civil Engineering Architecture, Beijing, 100044, China
This paper is focused on time-frequency feature parameters and genetic algorithm neural network techniques in fault diagnosis of railway rolling bearings. The time-frequency feature parameters for classification are extracted from vibration signals. However, the weak features of faults in rolling bearing are always immersed in noises of the environment, to solve this problem, Firstly, the wavelet analysis is used to filter and de-nosing and the time domain features are calculated. Secondly, the EMD (Empirical Mode Decomposition) method is used to decompose the signal into a number of intrinsic mode functions (IMFs), and then the IMF energy-torques could be calculated through the de-nosing signal. Finally, the genetic algorithm neural network is used for the classifications of the time-frequency feature parameters. The results of the time-frequency feature parameters and genetic algorithm neural network (GNN) show the effectiveness and the high recognition rate in classifying different faults of railway rolling bearing.