Validation assessment with uncertain model inputs
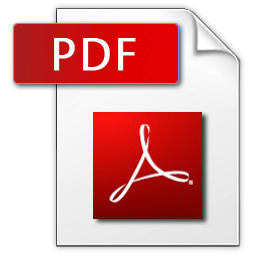
Liang Zhao, Zhanping Yang
Institute of Electronic Engineering, China Academy of Engineering Physics, 621900 MianYang, China
This paper presents a validation assessment method to measure the discrepancies between the model predictions and experimental observations under both aleatory and epistemic uncertainty. The model inputs considered in the paper are sparse point data or interval data, which leads to uncertain parameters for the distribution of the model inputs. A likelihood based method is used to represent the stochastic model inputs and it yields a single probability distribution which integrates the aleatory and epistemic uncertainty of model inputs. This representation of model inputs provides an advantage in computation efficiency for the conventional double loop sampling requirement in uncertainty propagation is collapsed into a single loop sampling. An area based validation metric is extended to compare the probabilistic model predictions obtained from uncertainty propagation with the empirical distribution function of the experimental observations, it reflects an objective quantification of the entire discrepancies between predictions and observations. The confidence interval for the validation metric, which just depends on the amount of experimental observations and confidence level is also developed. A numerical example is used to illustrate the proposed method.