Face super-resolution algorithm based on SVM-improved learning
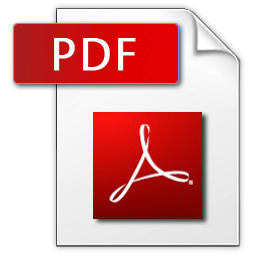
Jiali Tang1,2, Chenrong Huang3, Yijun Liu2, Honghui Fan2, Jianmin Zuo1,3
1 School of Mechanical Engineering, Jiangsu University, Zhenjiang 212013, Jiangsu, China
2 College of Computer Engineering, Jiangsu University of Technology, Changzhou 213001, Jiangsu, China
3 School of Computer Engineering, Nanjing Institute of Technology, Nanjing 211167, Jiangsu, China
As many other inverse problems, human face image super-resolution is an ill-posed problem. The problem has been approached in the context of example-based superresolution learning. However, these methods need to run though all the sample set, which results in high calculation load and image degradation because of mis-matching. In this paper, we propose a new face image superresolution algorithm based on Support Vector Regression (SVR) pre-classified learning. A Principal Component Analysis (PCA) based pre-process is used to select a subset of samples. Then the best-matching sample images are trained to ensure the content relevance between the sample patch and the input low-resolution image. Further improvement involves a combination of classification and SVR-based techniques. Therefore, experiment results show that the proposed algorithm gets better reconstruction performance and faster program running speed.