Research on cost-sensitive ensemble classification for mining imbalanced massive data streams
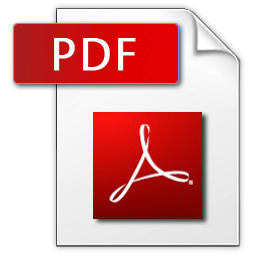
Yuwen Huang1,2
1Department of Computer and Information Engineering, Heze University, Heze 274015, Shandong, China
2Key Laboratory of computer Information Processing, Heze University, Heze 274015, Shandong, China
Abstract
The existing classifiers for massive data streams do not consider the imbalance distribution and cost factors, so this paper proposes the approach of the cost-sensitive ensemble classification for imbalanced massive data streams (CECIDS). Firstly, this paper gives the construction method for cost-sensitive ensemble SVM Classification, which is integrated by the classifiers with oversampling, sub-sampling and reconstituted sample space. Secondly, we propose a classifier method BL_KNNModelб which is based on KNNModel algorithm for imbalanced massive data streams. BL_KNNModel can detect the concept drift streams by using the variable windows size, which has lower time complexity. At last, the cost-sensitive ensemble classifier for imbalanced massive data streams is given, which has the virtue of high classification and lower time complexity. In addition, the cost-sensitive ensemble SVM algorithm is used to handle the confused instances. The experiments using both synthetic and real datasets show that compared to the other classification algorithms for imbalanced data streams, CECIDS has higher evaluating indicator and more excellence integrated learning curve.