Truncated aggregate homotopy algorithm for the least trimmed squares estimation in nonlinear regression
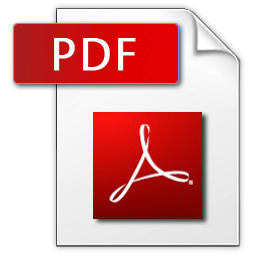
Yu Xiao1, Zhigang Yan2
COMPUTER MODELLING & NEW TECHNOLOGIES 2014 18(12A) 86-92
1School of Basic Science, East China Jiaotong University, Nanchang, Jiangxi, 330013, China
2School of Accounting, Jiangxi University of Finance and Economics, Nanchang, Jiangxi, 330013, China
In data regression, an important role is played by the least trimmed squares (LTS) estimate, which is less sensitive to the outliers than some other estimators such as the least squares estimator. However, estimating the LTS in nonlinear regression would be unimaginable expensive. For the data set with sizemand outliers , it would require nonlinear least squares regressions. To solve this problem, this paper studies the LTS solution from an optimization point of view, and proposes truncated aggregate homotopy algorithm to the equivalent min-min-sum programming. Numerical tests with comparisons to some other methods show that the new method is efficient