Estimating fundamental matrix from uncalibrated images
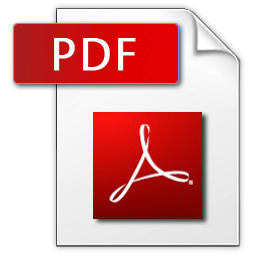
JiangmingKan, Chuandong Zhan, Shuo Feng,Wenbin Li
COMPUTER MODELLING&NEW TECHNOLOGIES 2014 18(12B) 595-602
School of Technology, BeijingForestryUniversity, Beijing, 100083 China
Estimation of the fundamental matrix plays a significant role in the field of computer vision. Two different approaches are presented to estimate the fundamental matrix from uncalibrated images: one is an improved iterative approach; the other an improved robust estimation. The improved iterative approach, utilizing the least-squares technique, makes use of several point matches to compute the initial fundamental matrix and weights and determines the computation loop by concerning the Euclidean distance between matched points and epipolar lines. The improved robust estimation extends the original RANSAC approach by removing outliers from the points set every five inner loops after being evaluated with corresponded scores to get the optimal points set and then estimating the fundamental matrix through the orthogonal least-square algorithm at each iteration. Experimental results that the improved iterative performs better when both the variance of Gaussian noises and the percentage of outliers are small. Results reveal that the proposed technique of removing outliers works successfully and fine, especially with a high level of outliers; and it is superior to the original RANSAC in terms of means and standard deviation on real images.