Depth Induced Feature Representation for 4D Human Activity Recognition
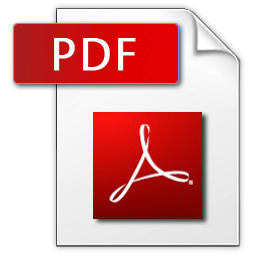
Runlin Zhao1, Yang Zhao2
COMPUTER MODELLING & NEW TECHNOLOGIES 2014 18(12C) 419-423
1Department of Computer Science and Technology, Yuncheng University, Yuncheng, China
2School of Automation, University of Electronic Science and Technology of China, Chengdu, China
Human activity recognition based on RGBD data has drawn considerable attention due to recent emergence of low-cost depth cameras. Essentially, human activities are composed by human bodies moving in four-dimensional space, (x,y,z,t). The traditional human activity recognition approaches usually ignore depth information thus degrading its discriminative performance. In this paper, our contributions are two-fold. First of all, we learn an Activity Depth Mapping (ADM) over each activity from training samples, where Activity Depth Maps are represented by Gaussian Mixture of Models (GMM) and encode depth distributions of activities. Second, we propose a novel feature representation, called Depth-Induced Multiple Channel STIPs (DIMC-STIPs), for activity representation with RGB-D data where both color and depth channels are available. The proposed feature representation is evaluated on the public dataset RGBD-HuDaAct and it remarkably improves the classification accuracy over state-of-the-art approaches.