Fault Diagnosis Model of Transformers Based on Neighborhood Rough Set and Relevance Vector Machine
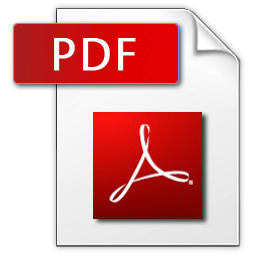
Chen Jialin1, Zhang Mingyu2, Duan Jiahua1
COMPUTER MODELLING & NEW TECHNOLOGIES 2014 18(12D) 117-120
1Yunnan Province Energy Investment Group co., LTD, Yunnan Kunming, 650021;
2 Energy Industry Development Institute of Yunnan Province Energy Investment Group co., LTD, Yunnan Kunming, 650021)
Transformers are important devices in power supply system. Since the testing data in fault diagnosis of transformers have features such as high dimension, uncertainty and incompleteness, existing fault diagnosis methods are incapable of dealing with the high-dimensional and abnormal data, and the diagnosis precision needs further improvement. This paper innovatively proposes a fault diagnosis model for transformers based on neighborhood rough set (NRS) and relevance vector machine (RVM). It integrates advantages of NRS in handling uncertain and incomplete information and RVM’s capability in dealing with high-dimensional data. Based on data provided by Yunnan Kunming Power Supply Company in fault diagnosis of transformers, case study has shown that the testing diagnosis rate of four classifiers in this model are 92.55%, 93.87%, 93.44% and 92.28% respectively, higher than the diagnosis precision of RVM, which indicates this model enjoys better diagnostic capability.