Cost-sensitive back-propagation neural network for financial distress prediction
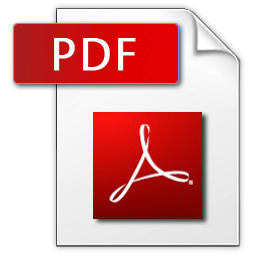
Wang Hongbao
COMPUTER MODELLING & NEW TECHNOLOGIES 2014 18(2) 259-264
College of Applied Foreign Language, Heilongjiang University, No. 74 Xuefu Road, Harbin, Heilongjiang Province, China, 150080
Financial distress prediction (FDP) models, which classify financially distressed companies from healthy ones, prevent market participants from suffering economic loss. In the process of FDP, the misclassification of type I error of the model incurs much higher cost than that of type II error. Most of the previous FPD models do not take the asymmetric costs into consideration. In this paper, cost-sensitive back-propagation neural network (CS-BPNN) FDP model is proposed for minimizing the cost of prediction error such that the loss of users of the model will suffer less. The performance of the model is evaluated by taking 180 Chinese listed companies as sample data and adopting 8 times of sampling to assess different misclassification costs and prediction accuracy. The experimental results suggest that the proposed approach helps to improve the prediction performance in asymmetric cost setup.