AN ABNORMAL USER BEHAVIOUR DETECTION METHOD BASED ON PARTIALLY LABELLED DATA
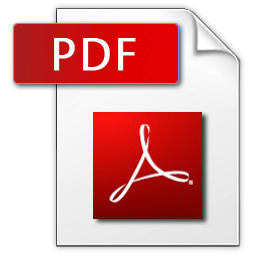
You Lu, Xuefeng Xi, Ze Hua, Hongjie Wu, Ni Zhang
School of Electronic and Information Engineering, Suzhou University of Science and Technology, Suzhou, P.R. China
Detecting abnormal user behaviour is of great significance for a secured network, the traditional detection method, which is based on machine learning, usually needs to accumulate a large amount of abnormal behaviour data for training from different times or even different network environments, so the data gathered is not in line with practical data and thus affects accuracy, and that increases overhead for data labelling. In light of these disadvantages, this paper proposes the detection method based on collaborate learning, it uses under-sampling method based on distance and distribution to generate training sample from imbalanced data, and semi-supervised learning method combined by ensemble classifying method to reduce demand for labelled data, it also uses differentiated member classifiers based on mixed perturbation method for collaborate training and selectively build ensemble classifier according accuracy to detect abnormal user behaviour. Experiments based on data from simulation and real network showed that this method can effectively detect abnormal behaviour and outperform traditional methods in several evaluating indicators.