TENSOR MODULAR SPARSITY PRESERVING PROJECTIONS FOR DIMENSIONALITY REDUCTION
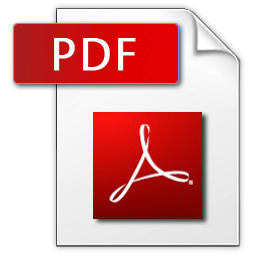
Mingming Qi1, 2, Yang Xiang1
1Department of Computer science and technology, Tongji University, Shanghai, 201804, China
2Yuanpei College of Shaoxing University, Shaoxing, Zhejiang 312000, China
In order to reduce the computational complexity and promote the classification performance of Modular Weighted Global Sparse Representation (MWGSR), Tensor Modular Sparsity Preserving Projections (TMSPP) for dimensionality reduction is proposed. The algorithm firstly partitions an image into several equal-sized modules and constructs these modules into a third-order tensor image; then, the algorithm makes module sparse reconstructions and some modules with less reconstruction errors are selected. These selected modules are recombined into a dataset with fewer dimensions and a new sparse reconstruction weight is gotten on the new dataset, which is denoted as the sparse reconstruction weight of original samples; finally, projection matrices are gotten with steps of tensor sparsity preserving projections on the reconstructed tensor images. The algorithm promotes the computational efficiency and the robust performance of sparse preserving projections on high-dimensional datasets. Experimental results on YaleB and AR face datasets demonstrate effectiveness of proposed algorithm.