SVM CLASSIFICATION OF HYPERSPECTRAL IMAGES BASED ON WAVELET KERNEL NON-NEGATIVE MATRIX FACTORIZATION
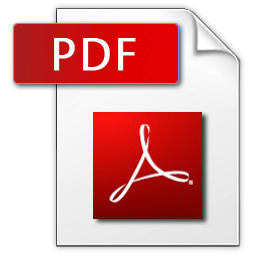
Lin Bai, Meng Hui
School of Electronics and Control Engineering, Chang'An University, 710064, Xi’An, P.R.China
This paper presents a new kernel framework for hyperspectral images classification. In this paper, a new feature extraction algorithm based on wavelet kernel non-negative matrix factorization (WKNMF) for hyperspectral remote sensing images is proposed. By using the feature of multi-resolution analysis, the new method can improve the nonlinear mapping capability of kernel non-negative matrix factorization. The new classification method of hyperspectral image data combined with the novel kernel non-negative matrix factorization and support vector machine (SVM). The simulations results show that, the method of WKNMF reflect the nonlinear characteristics of the hyperspectral image. Experimental results on Airborne Visible Infrared Imaging Spectrometer 220 bands data in Indian pine test site and HYDICE 210 bands hyperspectral imaging in Washington DC Mall are both show that the proposed method achieved more strong analysis capability than comparative algorithms. Compared with the PCA, non-negative matrix factorization and kernel PCA method, classification accuracy of WKNMF with SVM can be improved over 5%-10%.