THE EFFECTIVENESS OF USING METHODS TWO-STAGE FOR CROSS-DOMAIN SENTIMENT CLASSIFICATION
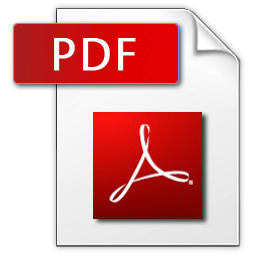
Hoan Manh Dau, Ning Xu
School of Computer Science, Wuhan University of Technology, China
Traditional sentiment classification approaches perform well in sentiment classification but traditional sentiment classification approaches does not perform well with learning across different domains. Therefore, it is necessary to build a system which integrates the sentiment orientations of the documents for every domain. However, this needs much labelled data involving and much human labour as well as time consuming. Thus, the best solution is using labelled data in one existed in source domain for sentiment classification in target domain. In this paper, a two-stage approach for cross-domain sentiment classification is presented. The First Stage is building a bridge between the source domain and the target. The Second Stage is following the structure. The study shows that the mining of intrinsic structure of the target domain brings a considerable effectiveness during the process of sentiment transfer. This is a typical mining approach comparing to previous approaches basing on information from the source domain to address the task of sentiment transfer, which does not depend on intrinsic structure of the target domain. Experimental results on sentiment classification with a two-stage approach indicate that the effectiveness outperforms other traditional methods.