Video target tracking with fisher discriminant dictionary learning
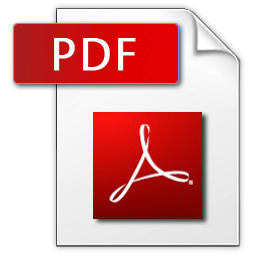
Jian-Feng Zheng, Ji Zhang
School of Information Science and Engineering, ChangZhou University, ChangZhou 213164, China
As one of the state-of-the-art tracking methods based on sparse coding, l1-tracker finds the target with the minimum reconstruction error from the target template subspace. But the high computational costs restrict its application in practical terms heavily. In this paper, we incorporate the discriminant information into original l1-tracker, and introduce it into the tracking framework, called FD2LT. In our framework, tracking is considered as a problem consisting of object location with dictionary learned in the last frame in generative tracking framework, training samples selection, and dictionary learning with fisher discriminant dictionary learning (FDDL). With our method, the dictionary is much smaller than that in original one, moreover, without loss of tracking performance (and even better in some scenarios). The discriminant power explored from the dictionary is used in generative tracking. Experimental results demonstrate the effectiveness and efficiency of the proposed tracking algorithm.