Cost-Sensitive learning on classification
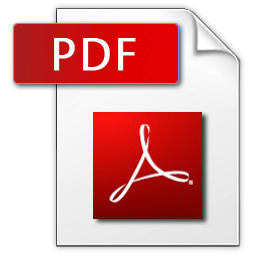
Qin Yang1, Changyao Zhou2
1School of Business, Sichuan Agricultural University, Dujiangyan 611830, Sichuan, China
2School of Resources and environment, Sichuan Agricultural University, Wenjiang 611130, Sichuan, China
Real-world predictive data mining (classification or regression) problems are often cost sensitive, meaning that different types of prediction errors are not equally costly. In this paper we propose a new algorithm for cost-sensitive classification in a multiple time series prediction problems. The fitness function of the genetic algorithm is the average cost of classification when using the decision tree, including both the costs of tests (features, measurements) and the costs of classification errors. The proposed model is evaluated in a real world application based on a network of satellite network map distributed in land spatial pattern evolution in Chengdu Plain. These satellite networks generate multiple time series data representing land spatial pattern. This study presents a new algorithm for cost-sensitive classification that deal with class imbalance using both recompiling and CSL. The method combines and compares several sampling methods with CSL using support vector machines (SVM). We build our cost-benefit model for the prediction process as a function of satellite network in a distributed land spatial and measured the optimum number of satellite network that will balance the expenses of the system with the prediction accuracy.