High resolution remote sensing image classification based on particle swarm optimization and support vector machine
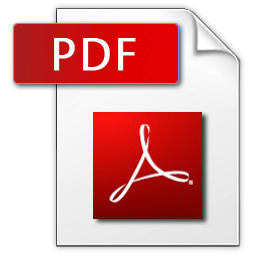
Buyi Li, Chongjing Deng, Shuang Li
COMPUTER MODELLING & NEW TECHNOLOGIES 2015 19(2B) 22-26
International School of Software, Wuhan University, Luoyu Road 37#, Wuhan, China, 430079
Many algorithms have been developed for image classification and support vector machine (SVM) is a kind of supervised classification that has been widely used recently. However, the accuracy of a SVM classifier heavily depends on the selection of a right kernel model and appropriate parameter. In this paper, a comparative analysis of the impact of four kernels (linear kernel, polynomial kernel, radial basis function kernel and sigmoid kernel) on the accuracy of SVM classifiers is conducted. Moreover, the Particle Swarm Optimization (PSO) is used to search for the optimum parameters for each kernel function in order to improve the classification accuracy of SVM classifiers. Our experiments for optimizing the kernel function parameters and assessing the robustness of SVM classifiers were carried out with classifications of QuickBird-2 images over Wuhan, China for monitoring urban land cover/land use information. The experimental results indicate that the polynomial kernel outperforms the other kernels in classifying high resolution remote sensing image. The sigmoid kernel performs worse than any other kernels. Our findings also suggest that selected parameter by PSO will improve the classification accuracy, especially for radial basis function kernel.