Dynamic multi-species coevolution large-scale optimize based on the fuzzy clustering and trust region
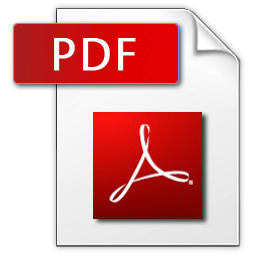
Xuhui Zhang
Department of Mechanical Engineering, Xi’an University of Science and Technology, No.58 Yatan Road, Xi’an 710054, China
Based on the above-mentioned studies, this article put the modified Fuzzy Clustering method into the particle swarm optimization, which solved the curse of dimensionality existing in the conventional algorithms. The large-scale parameter optimization method applied the modified Fuzzy C-Means method to clustering the large-scale dimension under the coevolution frame and achieved the valid dimension grouping. Later on, the dynamic neighbourhood topology multi-species particle algorithms divided the whole species into packets and constructed the subspecies sharing neighbourhood information, which improve the searching efficiency. The bring-in trust region could have the self-adapt adjustment for the particle optimization range, accelerate the optimizing speed, and decrease the iterations in the dead space. We use 20 standard large-scale testing functions for simulation. Compared with the top-ranked tournament algorithm, the mentioned algorithm achieved a better optimizing result in most functions, which surely laded the foundation of the large-scale neural network parameter optimization and the application in the control system.