Local reconstruction and local fisher discriminant based semi-supervised dimensionality reduction algorithm
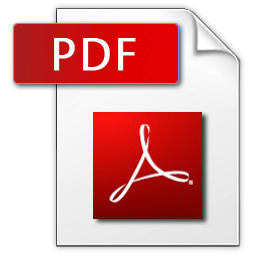
Xiangyang Chen
School of Electronic Engineering, University of Electronic Science and Technology, Chengdu, Sichuan Province, China, 611731
School of Mathematics and Computing Sciences, Guilin University of Electronic Technology, Guilin city, Guangxi Province, China, 541004
Local reconstruction and global preserving based semi-supervised dimensionality reduction (LRGPSSDR) algorithm gives no consideration to data locality when processing intra-class relationship and class relationship. Enhanced semi-supervised local fisher discriminant analysis algorithm (ESELF) also neglects locality of data manifold structure when maintaining data manifold structure. To address these problems, the local reconstruction and local fisher discriminant based semi-supervised dimensionality reduction (LRLFSDR) algorithm was proposed in this paper. It depicts significance of sample distance with an improved thermonuclear weight. In this way, intra-class relationship and class relationship of the same cluster attracts more attentions, thus enabling to shorten or widen intra-class distance or class distance firstly. Moreover, it uses idea of LLE algorithm to make neighbourhood linear reconstruction relationship of each point in low-dimensional space to be similar with that in high-dimensional space, which takes locality of data manifold structure into account. Test result confirmed that the proposed LRLFSDR algorithm is superior to other semi-supervised dimensionality reduction algorithms in classifying standard libraries like COIL20, Extended YaleB and CMU PIE.